From The Beatles to GenAI: The turning points shaping our future
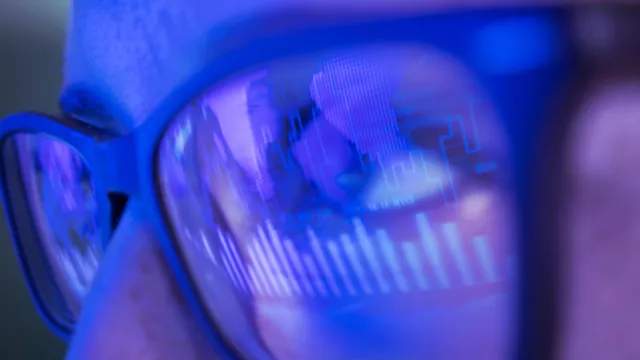
“Disclaimer: past performance does not indicate future returns” - the common small-print written in the footnote of any modern financial term sheet, advertisement and investment brochure.
In contrast, the adage of, “those that fail to learn from history are doomed to repeat it”, would suggest that past events can describe well the future.
The truth is somewhere in between: each generation experiences most of the things that previous generations have endured (bull or bear markets, natural disasters, geopolitics, …) punctuated by turning points from which the future takes a distinct path (powered flight, the transistor, The Beatles, …). These highlights are often recognized early on as important in their day and seem to appear ‘overnight’, and yet have taken years of development and formation to appear in our consciousness, while the lasting extent of their transformative power is not fully appreciated.
Generative AI (GenAI) fits the model described above, poised as it is to revolutionize treasury and capital markets by markedly altering decision-making processes for market professionals. From conversational finance to predictive analytics, AI is evolving from a mere assistant to becoming a crucial decision-making tool. To deliver on this potential, it is essential that financial institutions have access to high-quality data, upon which GenAI can infer connections, deliver insights and enable actions.
Data has never looked so good
Of course, ‘data’ has always been a recognized asset within financial markets. After all, market data vendors have made a good business in selling ‘tick’ data to banks for years, and regulators demand of banks to expend large efforts to protect ‘customer data’ in all its forms. Data has value. Yet with the development and access to GenAI, the latent value of high quality, granular data of all kinds, is growing. New connections and patterns emerge with seeming effortlessness, thanks to the computational crunch of Large Language Models (LLM), which lead to insights, forecasts, correlations, patterns and behaviours that can be monetized or actioned.
To truly unlock GenAI potential in areas such as workflows or user interactions, participants will need to expose data from existing, often disparate, applications. Existing data warehouses and data lakes are a good start, but not all data types flow into these locations today. For example, mission-critical treasury management systems (TMS) reporting, used as a key decision-making source, is localised in the application. Each reporting view evolves in content over time and is in essence a form of data in itself. Banks will want to expose and enable GenAI to reporting, to learn from these data time series, perhaps to indicate when reporting present key opportunities, or risks, that need action.
And we can see this trend in data centricity from another perspective. In a recent Finastra UK Treasury Forum event held in London, it was not uncommon to see attendees with job titles that included ‘Head of Treasury & Data’, as if to underline the fact that key banking functions such as Treasury management are coupled with data analytics beyond traditional cash management. Another perspective shows the US Bureau of Labor Statistics is projecting a growth of 36% over the period 2023-2033 in ‘Data Scientist’ jobs – a rate of growth much faster than average.
Timing is everything
And to frame the pace of change, let me share a personal experience.
In conversations with a large European bank a few years ago, the potential to leverage Treasury and Capital Market data as an asset without centralisation was explored. They wanted to allow data to persist in existing, traditionally siloed applications and systems and evoke an all-encompassing data layer that enabled citizen scientists access with low code/no code tools, to manipulate and co-mingle any and all data sets to extract deeper insights and monetization opportunities. Work efforts would pivot to more and more data-driven activities, supported with teams of employees, with the ability to question content across the business. Alas, the eventual scope and enterprise-level technologies that formed a viable target operating model was prohibitively costly for so ideal an outcome. The bank revised down their expectations and focused on more tactical approaches. Yet not a single thought was given to AI in the design thinking, as it seemed conceptual and remote, limited to labs or hyperscalers.
The lesson learned was not that the idea was wrong or flawed. The timing was just too early for the availability of tools that could inform a more agile approach. GenAI is opening up exactly this potential today.
‘Go big or go home’ – not any more
Already, expectations have shifted – due to what is actually available today and the constant development that is reaching users. Gone are the ‘tactical’ (local) vs ‘strategic’ (global) data management qualifiers of investment planning that would previously measure the importance of such architecturally significant projects. GenAI is dissolving the need to think in terms of coping with data here or there. Rather, the question posed now is how deeply any data set can yield added value. Having GenAI in some isolated deployment in operations, or in front office, or in reconciliations, is not a tactical approach; it’s just a smarter approach.
A financial institution’s success with GenAI will hinge largely upon that organisation’s ability to expose and manage large datasets and manage the quality of that data, with as broad a definition of ‘data’ as possible. In my view, it will not depend on any single, large data transformation strategy or enterprise-level approach. Starting small will bring immediate benefits of efficiencies, automation, and allow financial institutions to ‘connect the AI dots’ as deployments grow, unlocking even greater gains and data insights with the inevitably richer mesh of AI-infused technology that goes on to enable all of our businesses and marketplaces.
Outcomes lead to incomes
With anything really new, no contemporaries of the advance can ever have confidence in announcing any lasting impact and change of direction. Early adopters are perhaps the best gauge of what can be, and the flurry of use cases and ideas are put to the test to compete for status as the ‘killer app’ that dominates behaviours and markets thereafter. The real winners are those organisations that embrace a ‘fail fast’ attitude, don’t look for a perfect solution from the start and remain agile to change. GenAI gives businesses the space to experiment and learn, iterate and focus on good outcomes, all by interacting better with the data they already have. As ever, good outcomes lead to incomes, in the form of cost savings, added client value and margins. The GenAI revolution in financial markets is an extra dimension to existing business models, where incremental steps forward can have disproportionate returns for those who dare.