ESG & Generative AI – Clash of the brave new worlds (Part 1 of 3)
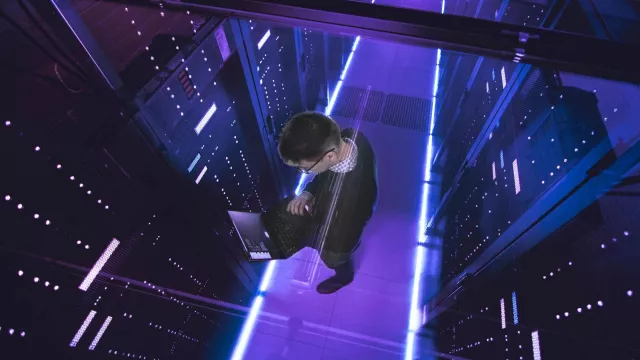
Part 1 of a new thought leadership series exploring the opportunity for ESG and AI. This first article sets the scene and outlines the potential for financial services.
In today’s rapidly evolving landscape, two critical issues dominate discussions worldwide: ESG and Generative AI. They have become the talking horses of not just the financial services industry, but almost every business worldwide in some capacity. In this mini-series we explore how these new kids on the block are shaping the future and how, when combined, they have unlimited potential to drive the future of sustainable financing.
Mark Zuckerberg, co-founder of Facebook, highlights the possibilities: “Generative AI is the key to solving some of the world’s biggest problems, such as climate change, poverty, and disease”. That’s all well and good, you may say, but what’s the context for financial services? McKinsey provides that context, reporting a global annual average spending need of $9.2 trillion on physical assets to aid the transition to Net Zero by 2050. So, clearly, we have both a compelling need for sustainable financing, and also now the ability to address many of today’s market challenges in an innovative way, leveraging the power of generative AI.
As with any good initial idea, the answer is often “show me the business case” and so here we delve into some collaborative examples.
Leveraging unstructured data
Finastra's State of the Nation Survey summarizes this challenge: “the potential for generative AI to extract information from unstructured data, including often-fragmented ESG data sources or siloed IT systems, is seen as a very compelling use case”. As organizations get to grips with the relatively new area of sustainable finance, they are quickly realizing that not only do they need a range of brand new data points, but that a lot of the existing data is scattered around multiple systems. Picture here a spider trapping a fly in every corner of its web and rushing around to pick up each morsel of food (or data in our case) individually; this is incredibly time-consuming, energy-sapping and inefficient.
Gen AI, however, can extract and consolidate the information from multiple sources instantly; and not only that, but it can provide rich insights into what the data is really telling us – all with minimal human effort. In the ESG context, KPMG's report highlights this need for automation; with 83% of companies surveyed still mainly using manual data gathering methods for their sustainability reporting.
Taking an example, let’s consider a company that wants to invest in a business operating in a different sector to them. At the touch of a button, AI can produce detailed analysis covering all aspects of the target company. In the ESG context, this may include compliance against sustainability rules for that industry or the physical carbon emissions associated with operating out of their business premises. Some companies such as CSRHUB can now even go as far as monitoring public opinion on a company’s overall sustainability credentials, using ‘big data’ to bring together thousands of unstructured data points into a consolidated view.
Leveraging the AI capabilities now available can provide deeper insights than we have ever had before, and the beauty is that it can be used in any context, from big corporations right down to the individual level.
Deterministic AI vs. Generative AI
Let’s start with a definition: deterministic AI involves the setting of rules, decision trees and logical reasoning. Given a particular input, these models will always produce the same output, with the underlying machine always passing through the same sequence of states.
Deterministic AI is therefore particularly useful in areas such as data analysis and calculations. It has been widely adopted within financial services due to the robust regulatory requirements of the industry. Strict rule paths are required for it to run accurately; however, one of the limitations is its ability to adapt to new scenarios and data sets.
This is where generative AI comes into its own. Through constant learning, Gen AI has the ability to evolve according to any new problem it is presented with. This is especially useful with unstructured data and in the ESG context where regulations are evolving rapidly. Of course, this comes with its own challenges, particularly in the ongoing ethical debate around AI’s usage and governance.
So what is the future of AI in financial services? While the two working harmoniously is the ideal future state, significant investment and testing will be required to get there.
Market context
In Europe it is estimated that, under the CSRD regulations, roughly 50,000 corporates across all industries will now have to report on the sustainability credentials of their business by 2025. The granularity of the data required will stretch beyond what many capture currently.
In years gone by, this would have been an incredibly onerous task for a business, and they would likely have needed to hire resource to comply. However, generative AI has arrived at just the right time to assist businesses with this reporting and reduce the bulk of the manual workarounds needed to fulfil these regulations.
Thinking back to our earlier spider metaphor, by employing AI appropriately, companies no longer need to rush around their network chasing their individual data morsels; they can wait comfortably in the centre of their web for generative AI to collate their data feast for them.
Some considerations I pose to the financial services market:
- Are we channelling our investment into AI appropriately and are we keeping it relevant to our end customers?
- Is our governance structure allowing the business to foster creativity whilst remaining compliant?
- Are we harnessing the power of AI to accurately monitor our ESG data and assist in reporting?
In part 2 of this series, we will explore these topics in the context of strategic decision making in financial services.